Identifying common transcriptome signatures of cancer by interpreting deep learning models, Genome Biology
Por um escritor misterioso
Last updated 03 abril 2025
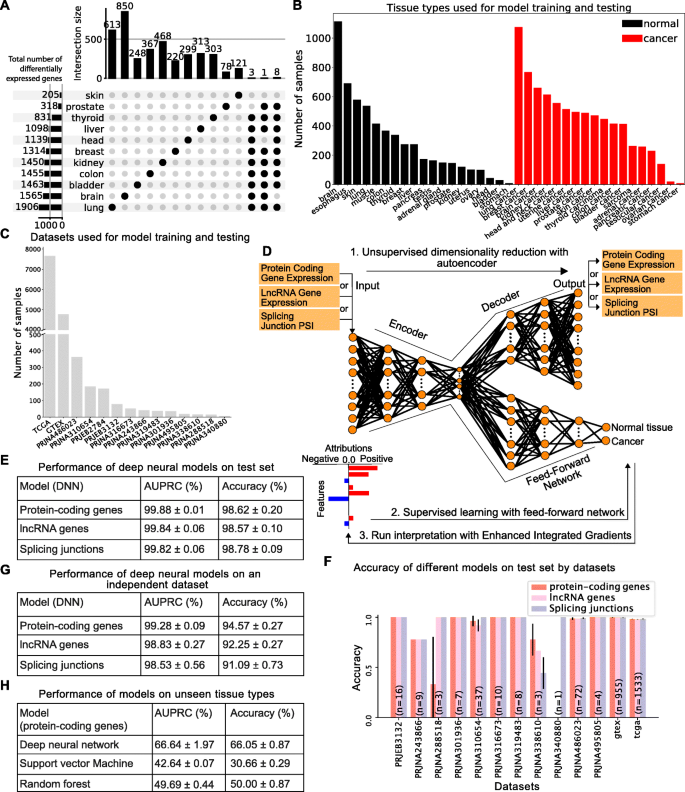
Background Cancer is a set of diseases characterized by unchecked cell proliferation and invasion of surrounding tissues. The many genes that have been genetically associated with cancer or shown to directly contribute to oncogenesis vary widely between tumor types, but common gene signatures that relate to core cancer pathways have also been identified. It is not clear, however, whether there exist additional sets of genes or transcriptomic features that are less well known in cancer biology but that are also commonly deregulated across several cancer types. Results Here, we agnostically identify transcriptomic features that are commonly shared between cancer types using 13,461 RNA-seq samples from 19 normal tissue types and 18 solid tumor types to train three feed-forward neural networks, based either on protein-coding gene expression, lncRNA expression, or splice junction use, to distinguish between normal and tumor samples. All three models recognize transcriptome signatures that are consistent across tumors. Analysis of attribution values extracted from our models reveals that genes that are commonly altered in cancer by expression or splicing variations are under strong evolutionary and selective constraints. Importantly, we find that genes composing our cancer transcriptome signatures are not frequently affected by mutations or genomic alterations and that their functions differ widely from the genes genetically associated with cancer. Conclusions Our results highlighted that deregulation of RNA-processing genes and aberrant splicing are pervasive features on which core cancer pathways might converge across a large array of solid tumor types.
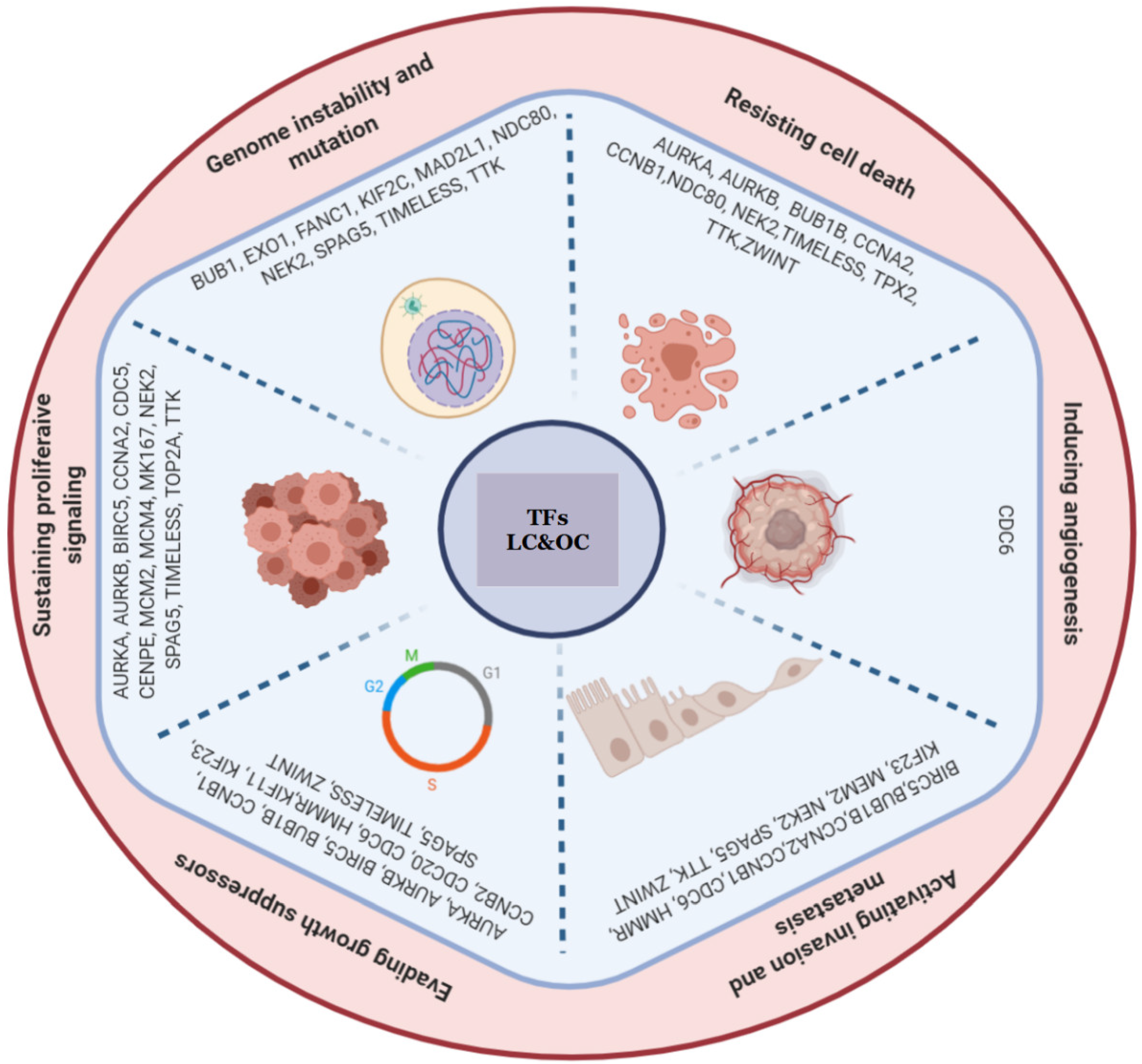
Biology, Free Full-Text
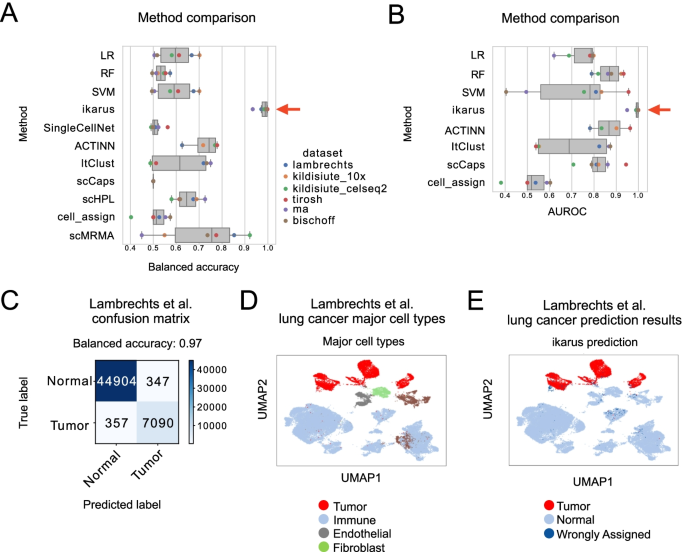
Identifying tumor cells at the single-cell level using machine
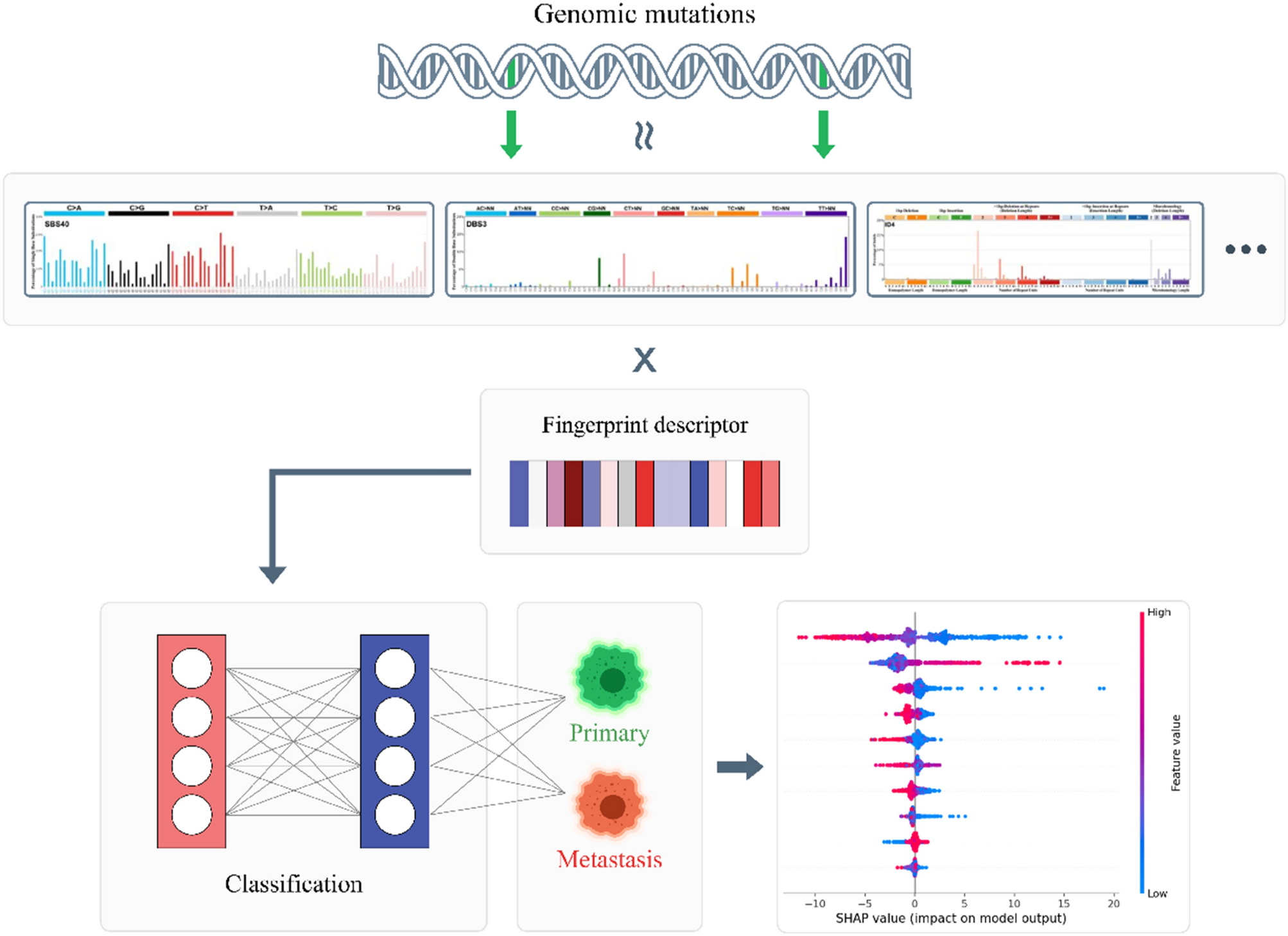
Deep learning model accurately classifies metastatic tumors from
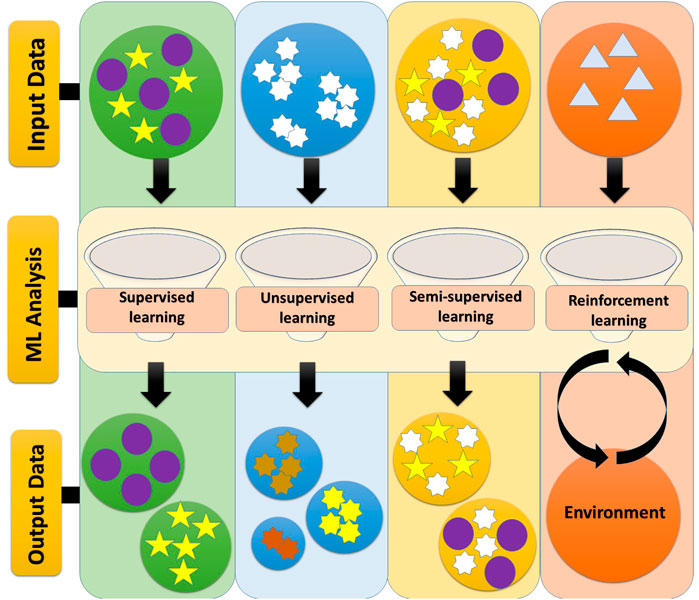
Frontiers Machine Learning: A New Prospect in Multi-Omics Data
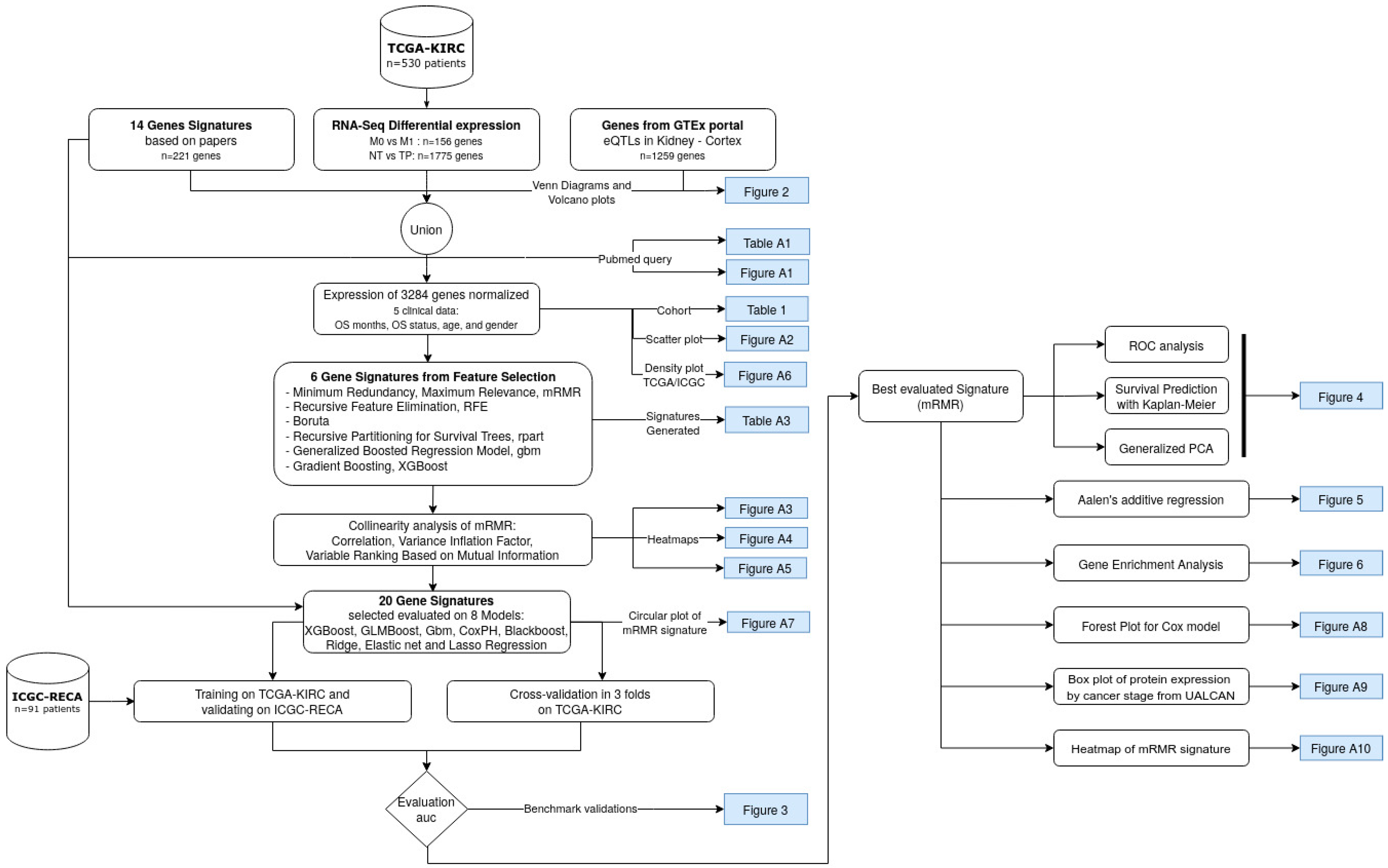
Cancers, Free Full-Text
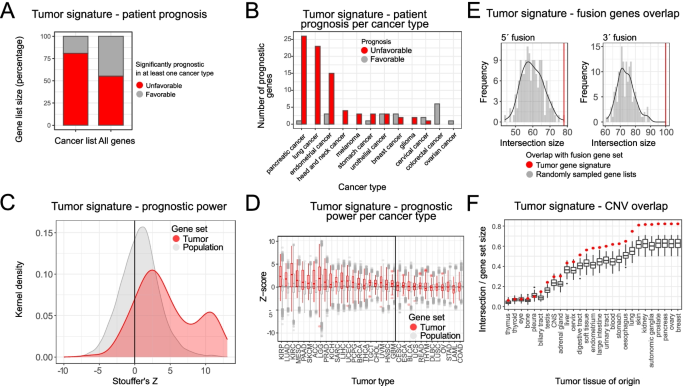
Identifying tumor cells at the single-cell level using machine

A Comparative Analysis of Single-Cell Transcriptome Identifies
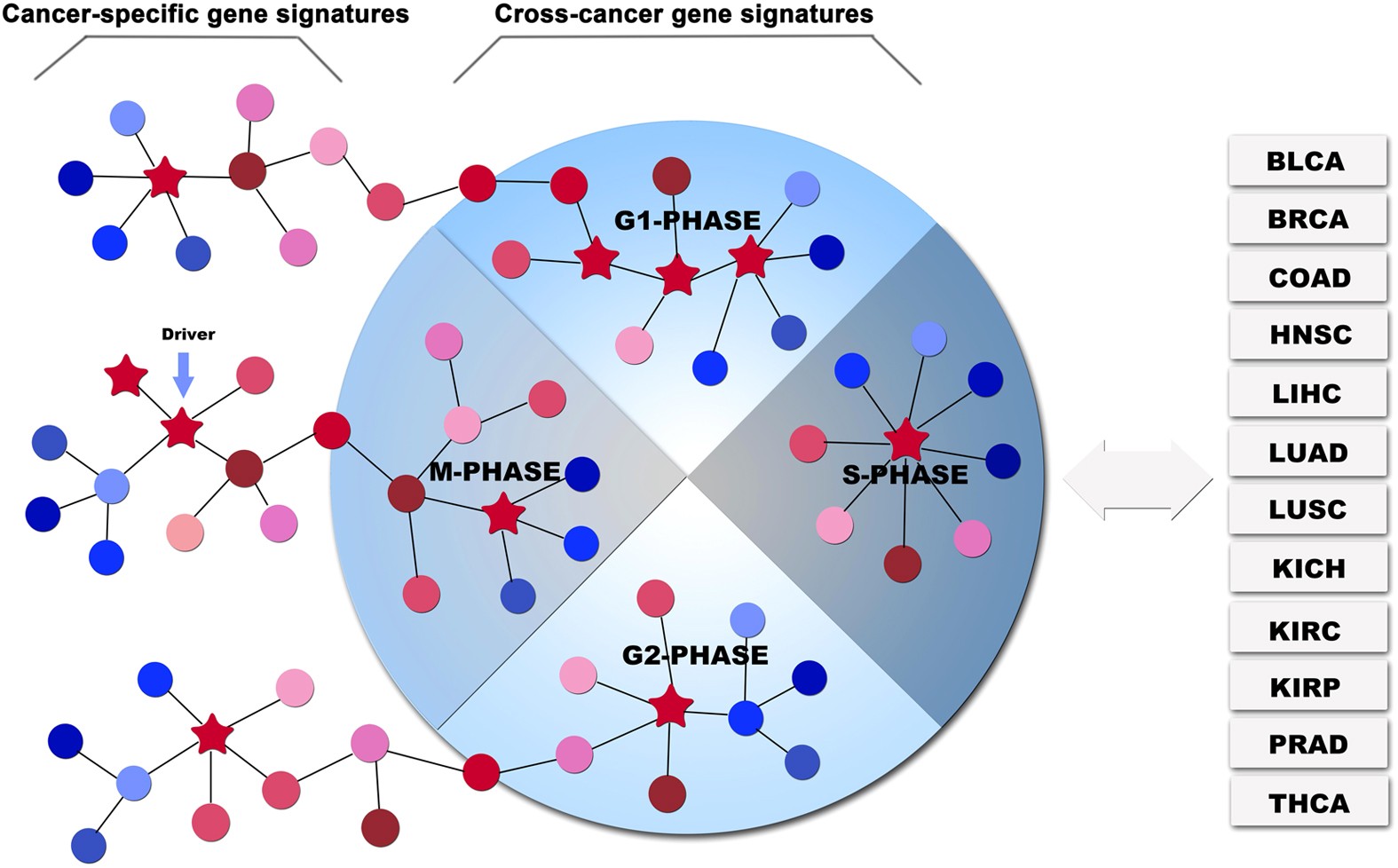
Large-scale RNA-Seq Transcriptome Analysis of 4043 Cancers and 548
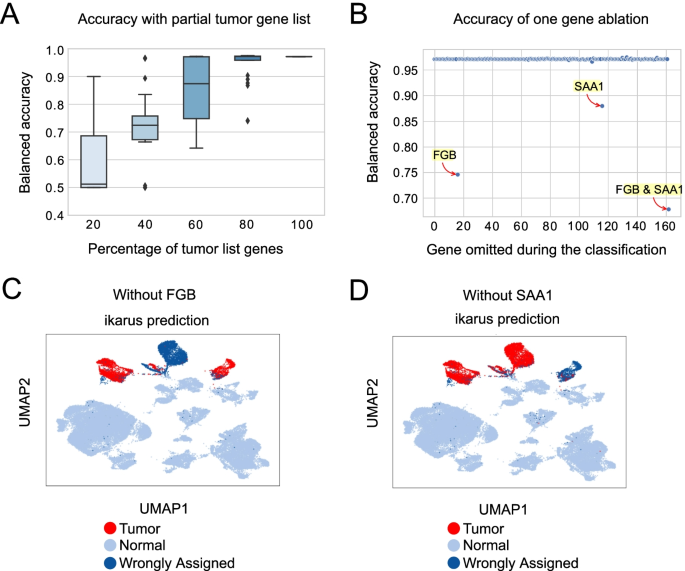
Identifying tumor cells at the single-cell level using machine
Recomendado para você
-
Death Incoming (level 367) #Android #Game #gameplay #gaming #apk03 abril 2025
-
SumDivide-best FREE block blasting puzzle game to test your brain::Appstore for Android03 abril 2025
-
Association of body mass index and waist-to-hip ratio with brain structure03 abril 2025
-
Subthalamic nucleus connectivity in binge drinkers and03 abril 2025
-
Carbon Monoxide Toxicity in Older Adults03 abril 2025
-
Fluid transport in the brain03 abril 2025
-
CBSE Class 12 Artificial Intelligence Question Paper 2023 with Answer Key (February 22, Set 4 - 367)03 abril 2025
-
A Cell Type Selective YM155 Prodrug Targets Receptor-Interacting Protein Kinase 2 to Induce Brain Cancer Cell Death03 abril 2025
-
Aiding and Abetting Anhedonia: Impact of Inflammation on the Brain and Pharmacological Implications03 abril 2025
-
Brain Test Level 361, 362, 363, 364, 365, 366, 367, 368, 369, 37003 abril 2025
você pode gostar
-
this used to be that backrooms Google maps thing. Now it's hell breaker bambi : r/FridayNightFunkin03 abril 2025
-
dream face reveal by k00pa Sound Effect - Meme Button - Tuna03 abril 2025
-
Dragon Ball Z Figura Majin Buu 18cm Busto Anime Figuras Kid Magro Fusão Buu GK Estatueta PVC Estátua Collectible Modelo Brinquedos Presente - AliExpress03 abril 2025
-
Black Friday Nintendo Switch Accessories: Best Deals Still Live on03 abril 2025
-
Jogo Da Cidade Da Skyline. 10 Cidades De EUA #1 Ilustração do Vetor - Ilustração de torre, boston: 2648294803 abril 2025
-
Butterfly Block Strike - Download Free 3D model by dchel12 (@dchel12) [64d51a8]03 abril 2025
-
Goldschmidt's grand slam lifts Cardinals to wild 6-5 win over Pirates03 abril 2025
-
Compra online de Grande floppa pelúcia bonito dos desenhos03 abril 2025
-
Clay Darren (taming.io) by quinneXoX on DeviantArt03 abril 2025
-
Fiscal de comemoração; VAR explicado: veja regras da Copa do Mundo feminina03 abril 2025