AlphaDDA: strategies for adjusting the playing strength of a fully
Por um escritor misterioso
Last updated 17 abril 2025
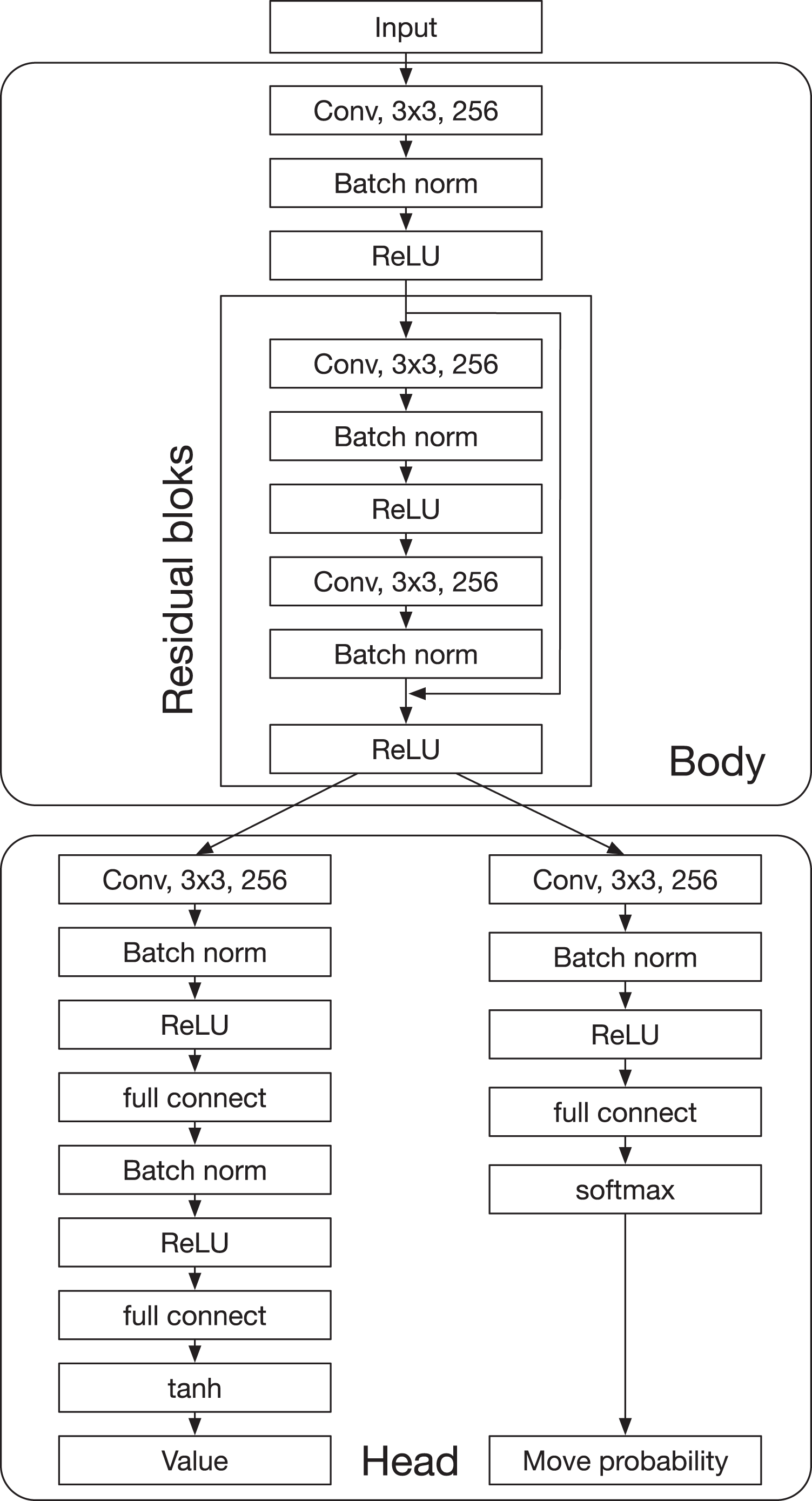
Artificial intelligence (AI) has achieved superhuman performance in board games such as Go, chess, and Othello (Reversi). In other words, the AI system surpasses the level of a strong human expert player in such games. In this context, it is difficult for a human player to enjoy playing the games with the AI. To keep human players entertained and immersed in a game, the AI is required to dynamically balance its skill with that of the human player. To address this issue, we propose AlphaDDA, an AlphaZero-based AI with dynamic difficulty adjustment (DDA). AlphaDDA consists of a deep neural network (DNN) and a Monte Carlo tree search, as in AlphaZero. AlphaDDA learns and plays a game the same way as AlphaZero, but can change its skills. AlphaDDA estimates the value of the game state from only the board state using the DNN. AlphaDDA changes a parameter dominantly controlling its skills according to the estimated value. Consequently, AlphaDDA adjusts its skills according to a game state. AlphaDDA can adjust its skill using only the state of a game without any prior knowledge regarding an opponent. In this study, AlphaDDA plays Connect4, Othello, and 6x6 Othello with other AI agents. Other AI agents are AlphaZero, Monte Carlo tree search, the minimax algorithm, and a random player. This study shows that AlphaDDA can balance its skill with that of the other AI agents, except for a random player. AlphaDDA can weaken itself according to the estimated value. However, AlphaDDA beats the random player because AlphaDDA is stronger than a random player even if AlphaDDA weakens itself to the limit. The DDA ability of AlphaDDA is based on an accurate estimation of the value from the state of a game. We believe that the AlphaDDA approach for DDA can be used for any game AI system if the DNN can accurately estimate the value of the game state and we know a parameter controlling the skills of the AI system.

Classification outcome in terms of error rate for given 3-mode tensor
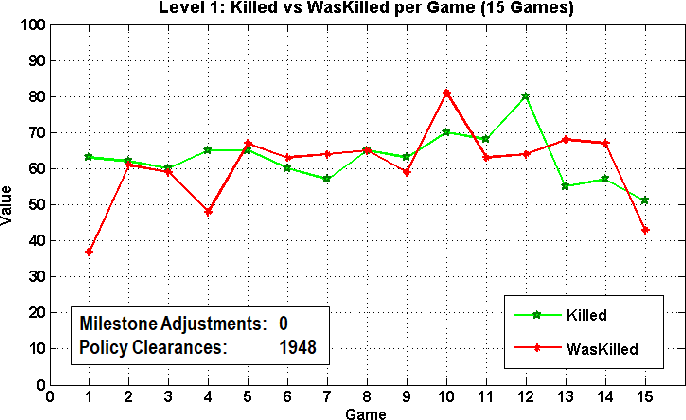
PDF] Skilled Experience Catalogue: A Skill-Balancing Mechanism for Non- Player Characters using Reinforcement Learning

Damage Output -Best Case (Static) Fig. 2. Damage Output -Best Case

arxiv-sanity

Total score of each game on the x axis.

User learning curve Download Scientific Diagram

藤田 一寿 (Kazuhisa Fujita) - マイポータル - researchmap
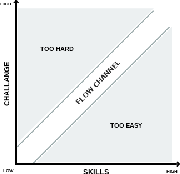
PDF] Dynamic difficulty adjustment through parameter manipulation for Space Shooter game

Average score in each game. DDA was active only in games 2 and 4.
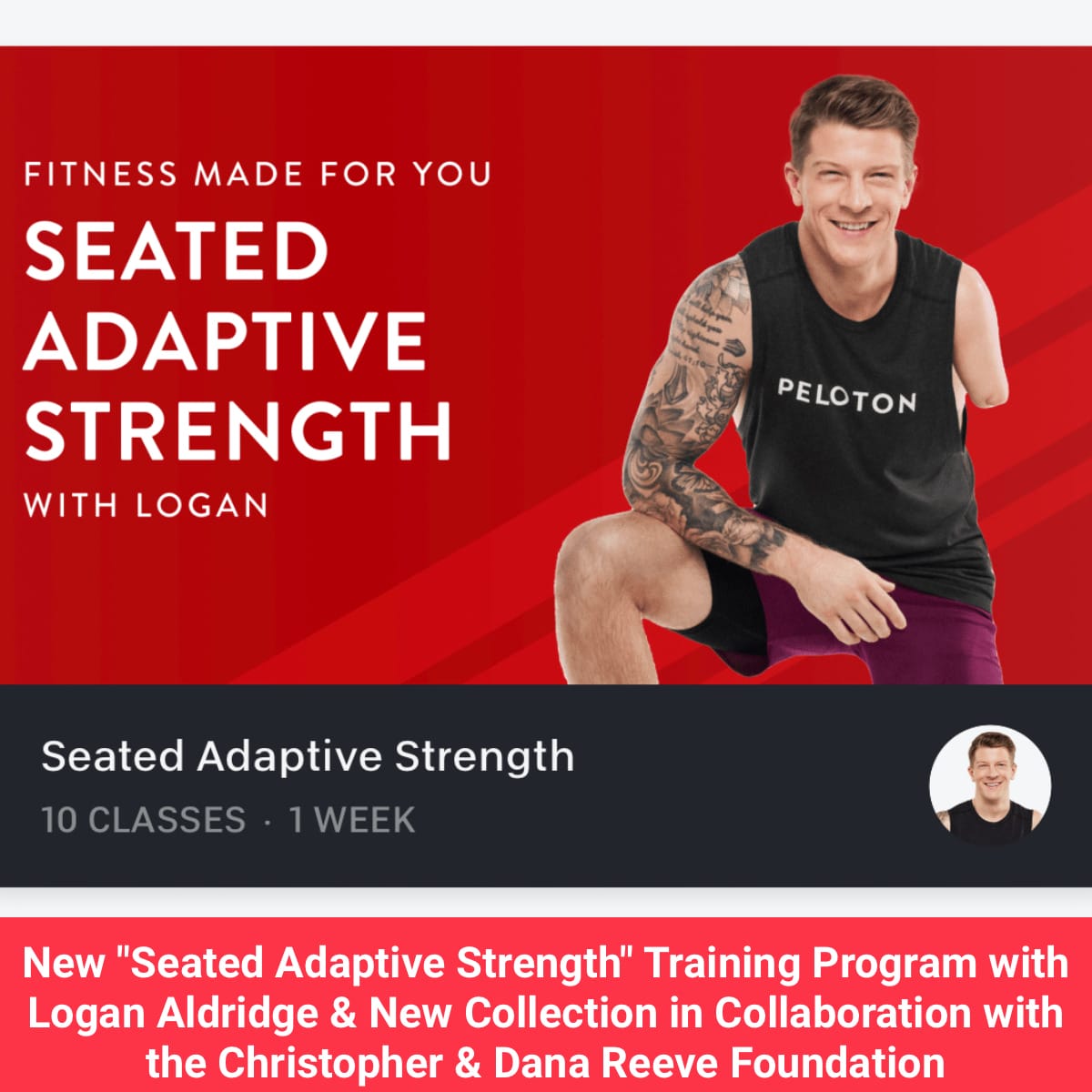
New Seated Adaptive Strength Training Program with Logan Aldridge & New Collection in Collaboration with the Christopher & Dana Reeve Foundation - Peloton Buddy

arxiv-sanity
An overview of Skilled Experience Catalogue.
Recomendado para você
-
RL Weekly 36: AlphaZero with a Learned Model achieves SotA in Atari17 abril 2025
-
Google AI Achieves Alien Superhuman Mastery of Chess and Go in Mere Hours - The New Stack17 abril 2025
-
AlphaZero: Reactions From Top GMs, Stockfish Author : r/chess17 abril 2025
-
GitHub - adepierre/Caffe_AlphaZero: Implementation of Deepmind's17 abril 2025
-
alpha-zero · GitHub Topics · GitHub17 abril 2025
-
Time manager Alphazero - Leela Chess Zero17 abril 2025
-
GitHub - CogitoNTNU/AlphaZero: An implementation of AlphaZero17 abril 2025
-
alpha-zero · GitHub Topics · GitHub17 abril 2025
-
xidong feng (@Xidong_Feng) / X17 abril 2025
-
From-scratch implementation of AlphaZero for Connect417 abril 2025
você pode gostar
-
QSMP Hiding from Coded Eggs by DoctorTrick17 on DeviantArt17 abril 2025
-
The Key to Better Bridge Master 2000 Cd-rom PC Computer 2004 Game Audrey Grant for sale online17 abril 2025
-
Manuel João Andrade - Cabo Verde, Perfil profissional17 abril 2025
-
The Series Factor17 abril 2025
-
The Devil is a Part-Timer manga Maou x Emi17 abril 2025
-
Gold Digger: Time Raft (Western Animation) - TV Tropes17 abril 2025
-
Explore the Best Mhaocxcanon Art17 abril 2025
-
Gibiate 1ª Temporada 2020 HDTV 1080p Completo Legendado17 abril 2025
-
you played yourself - Dave Chapelle (Fucking Up)17 abril 2025
-
Otaku 101: Guide to Watching Evangelion17 abril 2025